DeepSeek-R1 Series: Revolutionizing AI Reasoning Models with Reinforcement Learning
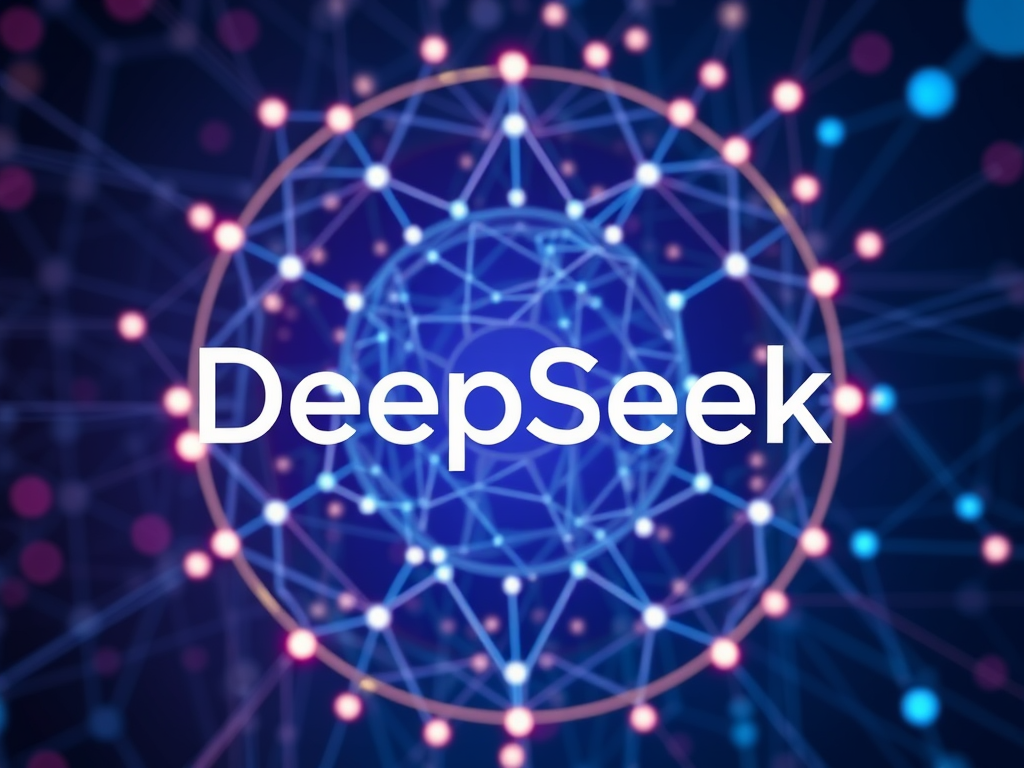
Introduction
The world of artificial intelligence continues to evolve at an unprecedented pace, and DeepSeek has just set a new benchmark in reasoning models. The DeepSeek-R1 Series, including DeepSeek-R1 and DeepSeek-R1-Zero, introduces groundbreaking advancements in reasoning, logic, and problem-solving capabilities. What makes these models remarkable is the innovative training methodology centered around large-scale reinforcement learning (RL), a departure from traditional supervised fine-tuning processes.
In this blog, we’ll dive deep into the unique features, performance comparisons, and open-source contributions of the DeepSeek-R1 Series and explore why these models are game-changers in AI reasoning.
DeepSeek-R1 is now available on ChatHub—give it a try!
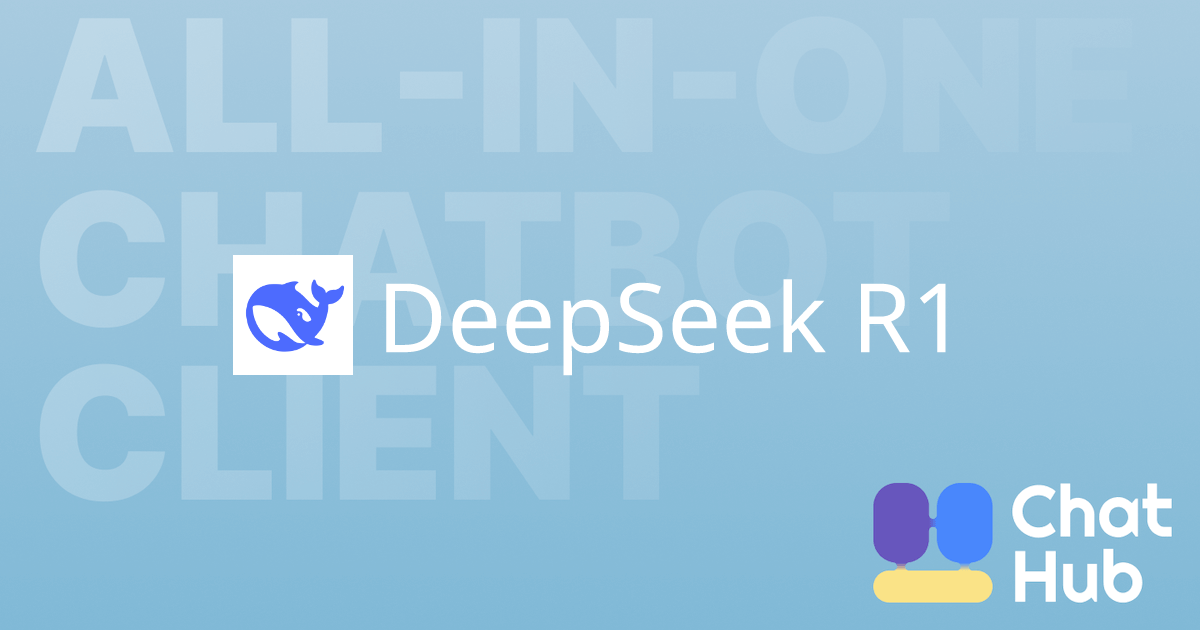
DeepSeek-R1-Zero: A Landmark in AI Training
Pure Reinforcement Learning: A Novel Approach
DeepSeek-R1-Zero stands as one of the most innovative AI reasoning models to date. It is the first large language model (LLM) to be trained entirely through reinforcement learning, without relying on supervised fine-tuning (SFT) or human-annotated datasets. This breakthrough validates the hypothesis that LLMs can develop powerful reasoning capabilities through reward-based RL signals alone.
Performance and Challenges
- Exceptional Reasoning Capabilities:
R1-Zero exhibits impressive self-reasoning abilities, often surprising researchers by autonomously developing unexpected yet highly effective problem-solving behaviors. Its reasoning in tasks involving mathematics, logical problem-solving, and even creative thinking rivals top-tier models in the industry. - Challenges to Overcome:
Despite its enhanced reasoning power, R1-Zero faces usability challenges. Generated outputs frequently include mixed-language content, particularly when prompted with multilingual tasks. Additionally, the readability of its responses can sometimes be inconsistent, making it less user-friendly for non-technical users.
DeepSeek-R1: The Balanced Hybrid
To address R1-Zero’s limitations while maintaining its strengths, DeepSeek introduced DeepSeek-R1, a hybrid model that merges reinforcement learning (RL) with supervised fine-tuning (SFT). This combination optimizes performance for both technical and general user preferences.
Let's take a look at the performance comparison between DeepSeek and other models.
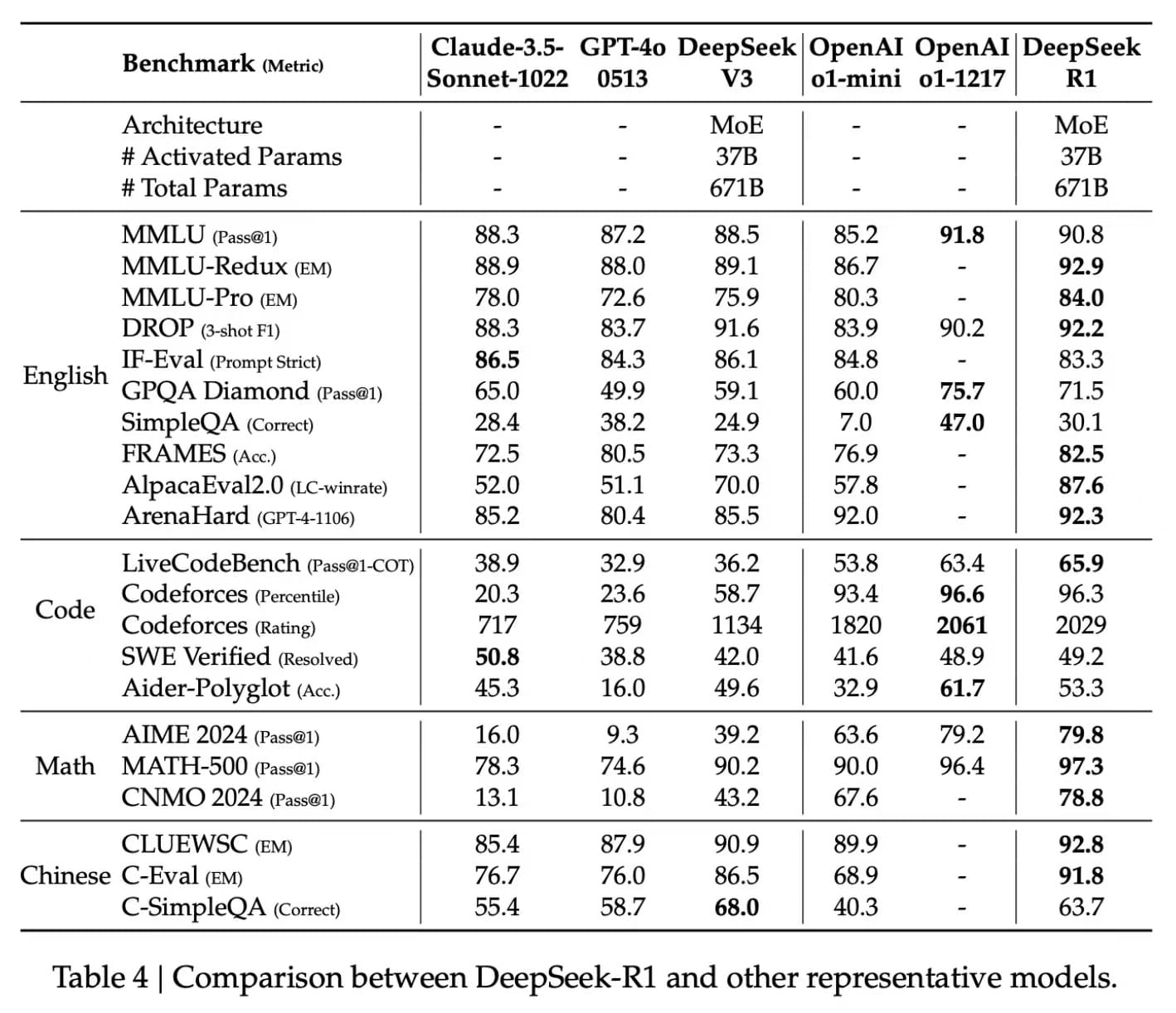
Balanced Training for Real-World Applications
- Cold-Start Supervised Training:
The training process begins with a "cold-start" stage, where the model is fine-tuned using a curated dataset designed for human alignment. This ensures the outputs are clear, accurate, and aligned with human expectations. - Reinforcement Learning for Advanced Reasoning:
R1 incorporates the same large-scale RL techniques used in R1-Zero to enhance its ability to tackle logic-intensive tasks, such as mathematical reasoning, coding, and scientific problem-solving. The RL process strengthens reasoning performance while reducing mixed-language issues observed in R1-Zero.
Performance Results
DeepSeek-R1 strikes a perfect balance between high reasoning accuracy and readable, human-aligned outputs. Whether it’s solving logic puzzles, writing accurate code, or answering scientific queries, R1 proves to be a powerful tool for reasoning-intensive tasks.
Performance Comparison: DeepSeek vs. OpenAI
DeepSeek-R1 vs. OpenAI o1
The DeepSeek-R1 Series positions itself as a serious competitor to OpenAI’s o1 line of models. On tasks that demand advanced reasoning, such as mathematics, coding, and natural language processing, the R1 Series performs on par with OpenAI’s o1 flagship models, making it one of the most advanced model series in the industry.
Surpassing OpenAI o1-Mini with Distilled Models
One of the most exciting aspects of the DeepSeek-R1 release is the open-sourced distilled models, which offer high performance at a smaller scale:
- Distilled Models:
Using the output of DeepSeek-R1, the team distilled six smaller models, including 32B and 70B parameter models, for community use. These distilled models exhibit comparable or superior performance to OpenAI’s o1-mini series, demonstrating DeepSeek’s ability to democratize cutting-edge AI capability.
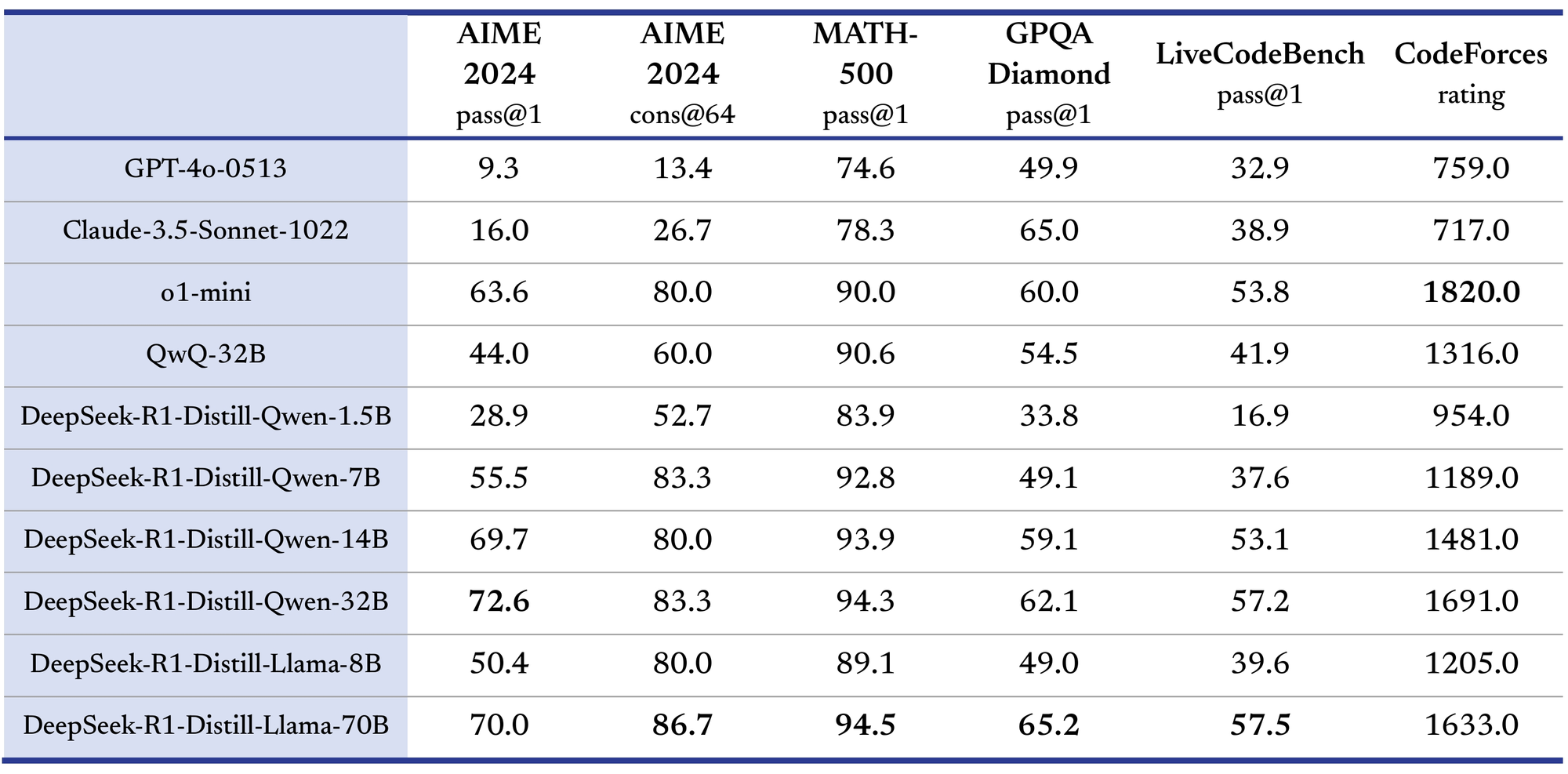
- Benefits for the Community:
By making these distilled models open-source, DeepSeek empowers researchers, developers, and startups to leverage advanced reasoning capabilities without the need for massive computational resources.
Innovative Training: What Sets DeepSeek-R1 Apart
Reinforcement Learning at Scale
Reinforcement learning plays a pivotal role in the R1 Series. By using RL to fine-tune reasoning-intensive tasks such as code generation and scientific problem-solving, DeepSeek models outperform peers in areas requiring step-by-step logic and clear solutions.
Challenges in Language Alignment
During training, researchers observed that multi-language prompts often caused language mixing in R1-Zero outputs. This was mitigated in R1 by integrating human-friendly supervised data in the initial phases, ensuring clearer and more structured outputs across various applications.
The Community Impact: Open-Source Contribution
DeepSeek stands out not only for its technical advancements but also for its commitment to open-source contributions. By releasing six smaller distilled models alongside DeepSeek-R1-Zero and DeepSeek-R1 (both 660B parameters), the company has made state-of-the-art AI reasoning models accessible to the wider AI research community.
For businesses, this means the ability to build advanced AI-driven applications without the enormous costs associated with proprietary models. Researchers, on the other hand, gain access to cutting-edge tools to further innovate in AI reasoning.
Applications of the DeepSeek-R1 Series
The DeepSeek-R1 Series unlocks potential across industries and domains. Here are a few key areas where these models excel:
- Mathematics and Logic:
Solve complex equations, perform rigorous proofs, and tackle logic puzzles with ease. - Code Generation:
Generate highly accurate code snippets, debug, and improve existing codebases. - Scientific Research:
Answer precise scientific queries, assist in hypothesis generation, and create structured solutions for defined problems. - Natural Language Understanding:
Provide human-friendly, clear, and concise responses to general queries, making them versatile for conversational AI or customer support.
Conclusion
The DeepSeek-R1 Series is a groundbreaking step forward in the AI reasoning landscape. With innovative reinforcement learning techniques, a hybrid approach combining SFT and RL, and unmatched performance against industry leaders like OpenAI, DeepSeek has solidified its position as a leader in AI innovation.
Moreover, the open-source release of distilled DeepSeek models democratizes access to advanced reasoning capabilities, paving the way for AI-powered tools and applications that were once exclusive to deep-pocketed corporations.
As we look to the future, the DeepSeek-R1 Series raises the bar for what reasoning models can achieve, both in performance and accessibility.
FAQs
- What is DeepSeek-R1-Zero?
DeepSeek-R1-Zero is the first AI reasoning model trained purely with reinforcement learning, offering cutting-edge reasoning abilities without relying on supervised fine-tuning. - What makes DeepSeek-R1 different?
DeepSeek-R1 combines supervised fine-tuning and reinforcement learning to balance powerful reasoning capabilities with user-friendly output. - Are the models open-source?
Yes! DeepSeek released six smaller distilled models (32B and 70B) as open source, along with R1-Zero and R1. - How does it compare to OpenAI o1?
The R1 Series performs on par with OpenAI o1’s flagship model and even surpasses the o1-mini series with its distilled versions. - Who can benefit from these models?
Businesses, developers, researchers, and startups can leverage these models for logic-intensive tasks, coding, scientific research, and more.
With the DeepSeek-R1 Series, the future of AI-powered reasoning is here—and it’s open to everyone.